Sometimes in A/B testing, it’s obvious what you need to test.
A full-screen takeover vs. a pop-up.
And sometimes, minor changes in design, text, CTA or timing make a drastic impact on engagement and conversion.
An image with a lot of negative space around it vs. a closeup.
Maybe that seems minor… but is it?
Luckily for digital marketers, you have the ability to hypothesize and A/B test to ensure the correct course of action.
Just the phrase “A/B test” tends to bring a negative connotation with it and we feel your pain. It takes long enough, usually, to get the first version of something up… so entertaining the idea of building an alternate version just to test seems daunting.
The good news is, your A/B tests don’t have to be anything crazy – even minor tweaks can produce big results.
Testing ensures that even when you aren’t sure what to do you can still act. Don’t worry about if your test doesn’t confirm what you wanted it to – all data is good data.
My team has made ‘all data is good data’ our guiding star. By following that star, giving ourselves room to fail, and iterating on what our testing tells us, we are able to discover and build big wins for our clients.
Let’s take a look at this successful Shopify pop-up test!
The 300% conversion increase
A recent big win for our team and our managed services was a 300% increase in conversion rate on a welcome pop-up!
That’s right. By iterating on design and following core principles we were able to create a winning variant that performed almost 300% better than the original.
Want to know how we did it?
Let’s take a look at what we started with:
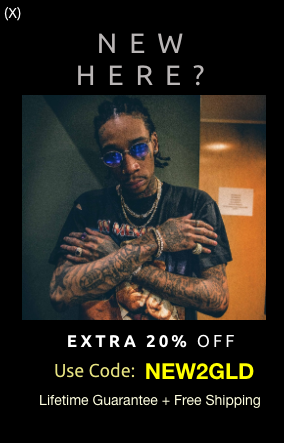
This was a pop-up running on the desktop for Shopify Plus client, The GLD Shop. The dimensions were small which worked great for mobile but was not so great for the desktop experience.
For some marketers the need to give this promotion a fresh design would be a no-brainer, right?
It’s actually not that obvious…Let’s look at the data:
The numbers
Engagement Rate: 20.6%
Conversion Rate (based on engagements: 11%
Is that new design still a ‘no-brainer’?
Most people would probably say ‘no’. After all, those are really solid numbers and no one wants to mess with good numbers. Still, the aesthetics of the site and the on-site experience had outgrown this promotion and ‘good’ numbers aren’t ‘great’ numbers.
This is the type of scenario testing is made for.
A higher-converting design we built
I’m sure you’re wondering what kind of massive, brilliant, clever thing we did to push conversions up by 300%. Surely the only way to get such massive improvements is to use some kind of cutting edge or experimental design, right?
Let’s take a look:
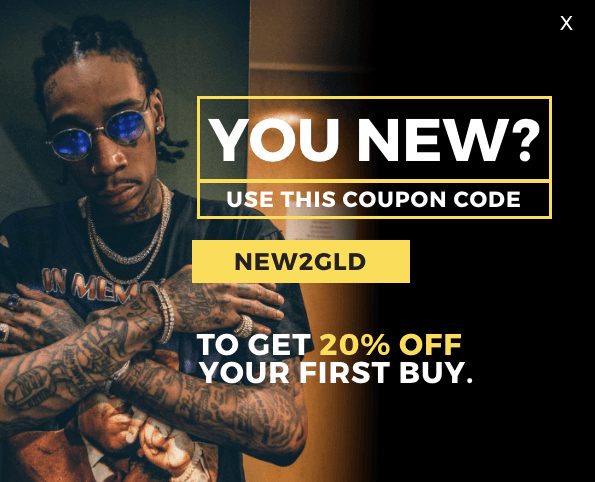
Here’s a breakdown of what we did:
- Larger, clearer image.
- Overall the pop-up is much bigger, roughly 3x as large
- The offer stands out via contrasting colors
- We got rid of generic, non-promotion specific text (lifetime guarantee + free shipping)
The above list is a great go-to checklist of elements of your promotions to look at when thinking about testing a redesign. These items tend to be really efficient in terms of time-management and help produce clear, exact deliverables (which we’re sure will help your project managers out).
Elements we didn’t change
It should be noted there are things we didn’t change about the original pop-up design:
- The discount offer is exactly the same (when A/B testing you should be testing the same discount offer. This is a huge variable and should be tested only ever in isolation)
- The image itself is the same (just much larger, much higher quality)
- The copy itself is also mostly the same. The largest copy change is embedding the discount into contextual language. Contextual language is great for removing questions from your offers.
Takeaways for your next A/B test
Here are some good lessons you can learn from this test.
- Pop-ups that do not match the aesthetic of the rest of your site will not convert as well. Always, always, always stay on-brand.
- If you have high-quality images, use them.
- Contrasting colors on your promotional focal points (where you want people to look/interact) still works very well
The most important lessons to take away from this test?
Always test and all data is good data!
Best practices exist for a reason – they give you a starting point, but it’s critical to keep in mind that best practices try to paint with a broad brush. They can and do miss things that only a data-driven testing cycle will reveal.